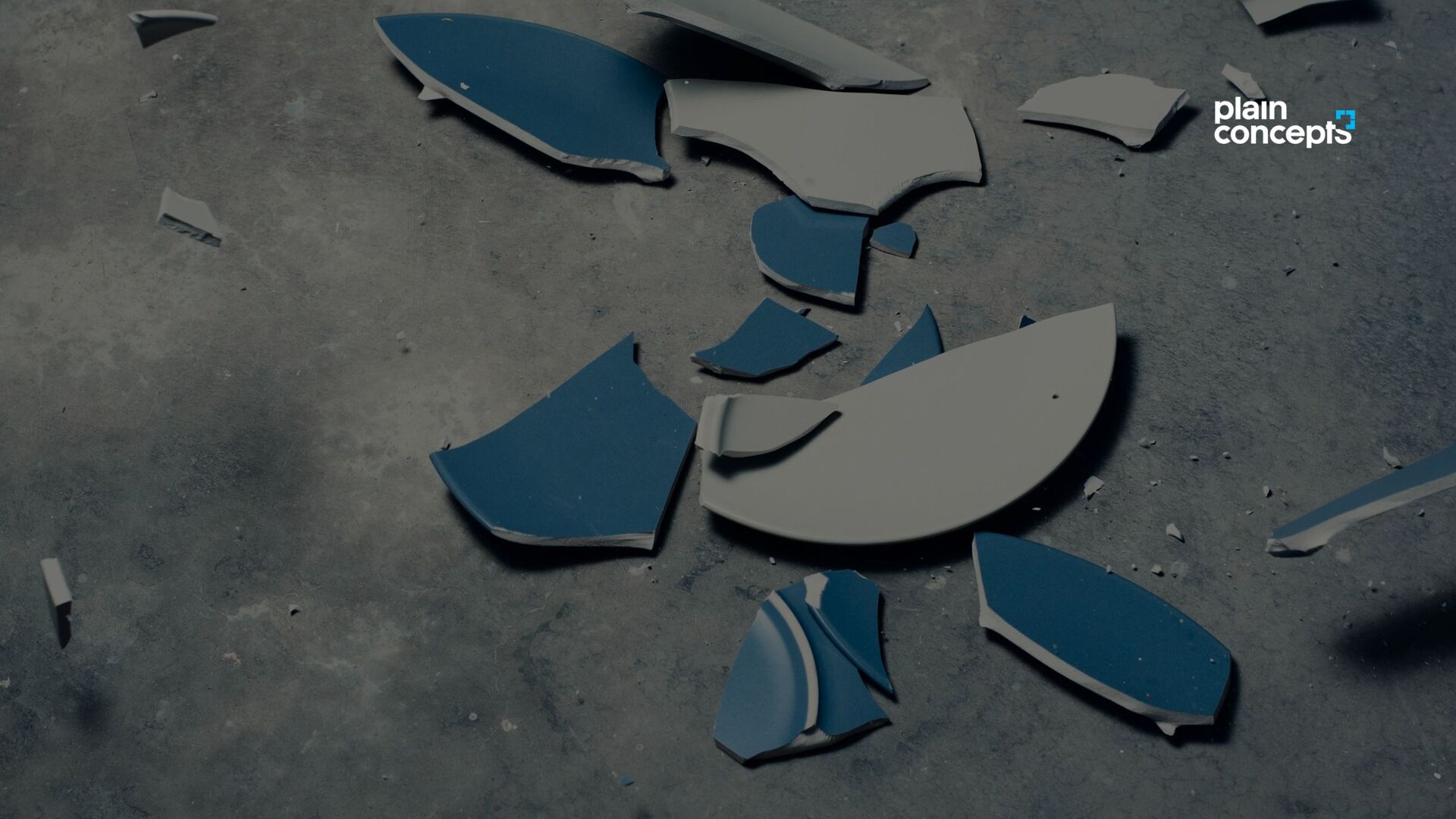
Why AI adoption fails in business: Keys to avoid it
- Reasons why many companies fail to introduce AI
- Top mistakes companies make when creating an AI strategy
- Failure to adopt a change management strategy
- Overestimate the capabilities of AI
- Failure to test and validate
- Ignoring ethical and privacy implications
- Neglecting data strategy
- Allocating inadequate resources
- Treating AI as a single project
- They forget about scalability
- Neglect infrastructure requirements
- Inadequately integrating with existing systems
- How to implement a successful AI strategy?
Artificial intelligence has been a turning point in the way of doing business for many companies globally. However, there are many cases that fail when it comes to introducing this technology into their processes and teams.
Having a solid AI strategy is a non-negotiable necessity to achieve a competitive advantage and results that really add value to the company. We analyze the most common mistakes that companies make when planning and implementing their AI strategy and give you the keys to avoid making them.
Reasons why many companies fail to introduce AI
According to a study by MITSloan, despite all the use cases that AI and ML can offer industries, the data reveals that 70% of companies say that AI has a minimal impact on their business and 87% of projects never go into production.
These are very worrying figures and reveal the huge problem, as well as the huge missed opportunities, of not having the right AI strategy in place and tailored to each case.
94% of business leaders agree that AI is critical to success in the next five years, but the reality is that most companies are lagging far behind.
Given these facts, one of the keys to avoiding the low level of success is to initiate AI projects that focus on business value. In addition, there are four main reasons why these projects fail: unclear business objectives, poor data quality, lack of collaboration between teams, and lack of talent.
Poorly defined commercial objectives
AI is a very powerful technology, but if we do not establish well the business problems our company has, as well as the objectives, it will be very difficult to be successful.
The important thing is to first determine and define the problems and then decide if and how an AI solution would help solve them. This is key to saving unnecessary time and costs.
Poor data quality
Data is one of the most precious assets for a company, so before starting any AI project, a good data governance strategy must be in place to ensure the availability, quality, integrity, and security of the data to be used in the project.
Working with outdated, biased, or insufficient data will waste resources and lead to failure. Therefore, ensure that you have sufficient and relevant data from reliable sources that represent business operations, are correctly labeled, and are suitable for the AI tool to be implemented.
Lack of cross-team collaboration
Having a data science team working in isolation on an AI project is a breeding ground for failure. To be successful, it requires collaboration between data scientists and engineers, IT professionals, designers, and business line professionals.
Practices such as DataOps and MLOps help bridge the gap between different teams and put AI systems in place at scale.
Talent shortage
This is a point that is not usually easy for companies to solve. It is one of the biggest challenges for companies, and that is that skilled data science professionals are in short supply.
Without a team with the right training and experience, there is less chance of achieving good results. Therefore, it is less costly, in terms of time and money, to opt for hiring a technology partner to help with business objectives and scale of operations.
Top mistakes companies make when creating an AI strategy
We have already looked at the main reasons why AI projects fail, but what are the main mistakes companies make when starting out with their AI strategy?
Failure to adopt a change management strategy
Many companies are unaware that adopting AI is not just about integrating new technology into existing processes. It requires a comprehensive change in the culture and operations of the entire organisation.
Clear and transparent communication about the adoption process will help alleviate fears or misconceptions and facilitate the change process.
Overestimate the capabilities of AI
Technology is a very powerful tool and can become our best ally, but not by magic. This belief leads to unrealistic expectations and disappointments, so we need to be aware of its limitations and how to approach it.
Models must be adjusted and refined, they cannot be expected to work 100% from minute one of their implementation.
Failure to test and validate
Failure to adequately test and validate AI systems can lead to inaccurate results, system errors, or serious damage.
AI systems are complex, so companies must plan for rigorous testing and validation to ensure safety, accuracy, and reliability.
Ignoring ethical and privacy implications
One of the biggest concerns with AI is ethical and secure solutions. Ignoring this can lead to risks that can damage a company’s reputation and cause legal complications.
This must be addressed proactively by incorporating transparency, fairness, and privacy safeguards into AI systems.
Neglecting data strategy
Without data, there is no AI, and neglecting the enterprise data strategy can deprive AI systems of the crucial information they need to function properly.
Therefore, companies need to plan very well how they collect and store data and how they will ensure that their data is organized, accessible, and of high quality.
Allocating inadequate resources
Make no mistake, the adoption of AI requires substantial investment in technology, talent, data and infrastructure. This will bring far more benefits than costs, but companies often underestimate these costs, resulting in insufficient resource and budget allocation.
As a result, AI initiatives are often unable to grow, failing to reach their potential or failing.
Treating AI as a single project
A good AI strategy is not a set-and-forget process. It requires ongoing maintenance, data updates and adjustments to adapt to new environments.
Companies that treat AI as a one-off project rather than a changing and growing initiative find that their systems become obsolete or ineffective. Continuous improvement will be the key to circumventing this, as well as allowing them to remain relevant and accurate.
They forget about scalability
Many companies test AI projects on a small scale, without considering the possibilities of scaling up these efforts.
Make no mistake, starting small is a good approach, but considering the scalability of projects from the outset helps avoid bottlenecks and inefficiencies in the future.
Neglect infrastructure requirements
Inadequate infrastructure can lead to performance issues and limitations in the implementation of advanced AI models.
This can compromise the efficiency and reliability of enterprise AI applications, leading to project failure and lost project investment.
Inadequately integrating with existing systems
Poor integration can result in ineffective Machine Learning applications, reducing efficiency and causing disruption to business processes.
This can result in wasted resources, as well as hinder the advancement and acceptance of enterprise AI in the organisational ecosystem.
How to implement a successful AI strategy?
AI is a journey that requires clear objectives, a deep understanding of our company’s capabilities, and an ongoing commitment to testing, privacy, talent, data strategy, and scalability.
A good AI strategy helps organizations address the complex challenges associated with AI implementation and define their goals. Regardless of the type of processes or applications you want to achieve, having a well-defined purpose and plan will ensure that AI adoption aligns with broader business objectives.
This alignment will be the key to extracting meaningful value from AI and maximizing its impact and results. It will also be critical to have a roadmap to address the challenges, develop the necessary capabilities, and ensure a strategic and responsible application of AI into the fabric of the organization.
At Plain Concepts we have more than 10 years of experience creating tailor-made solutions for our clients and can help you solve technological, informational, cultural, and organizational challenges. We will define together your strategy gradually and with tangible benefits.
We provide you with 4 main services to help you achieve an AI-driven company:
- AI Adoption Framework: discover, learn, identify, and define relevant high ROI use cases and new potential ones within your new strategy to be an AI-driven business.
- AI Centre of Excellence: develop a tailored AI strategy for your business. Customise and apply workflows, patterns, and communications to deliver high-value AI at speed.
- Generative AI Adoption Framework: we help you explore this new technology, identify how to use great language models, and understand their impact on your business model.
- MLOps Assessment: get your POC into production. We will standardize and streamline the Machine Learning lifecycle.
We’ll make sure your projects reach production. You won’t be left with ideas that are easy to implement, but with little impact on the business, where AI is not differentiating. We help you unlock the full potential of AI!