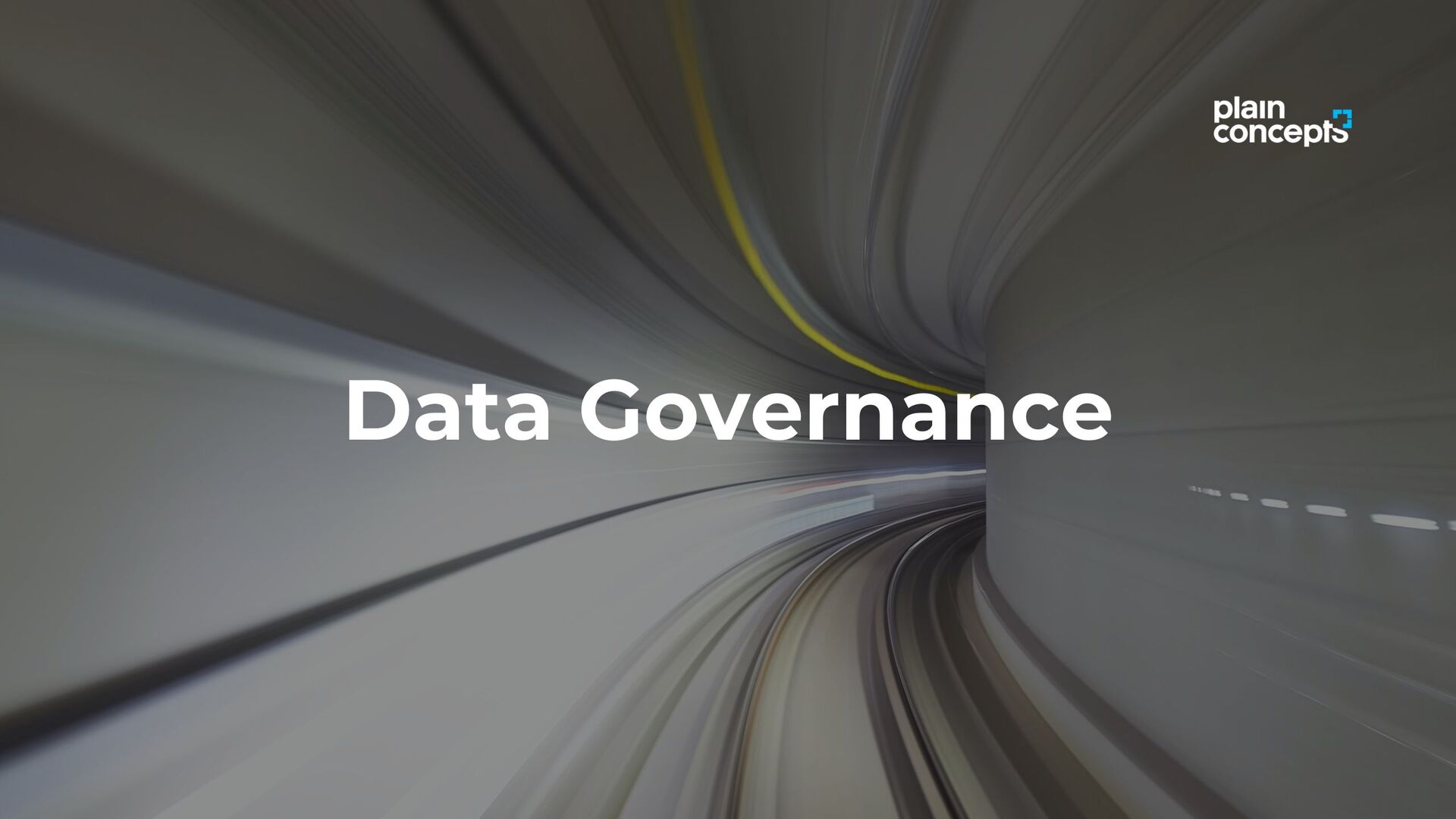
The importance of Data Governance in the digital age
Data facilitates better and more informed business decision-making, so as that data starts to grow, we can benefit from it in different types of business processes. But only if you do it the right way.
The more assets you collect, the more data-related problems you can encounter. Without good data governance, it is impossible to know when data enters our system, where it comes from, or who uses it. This can lead to poor data quality and reduced stakeholder confidence. We look at the importance of having a good data governance strategy and how to implement it in your organization.
Data Governance Objetives
Data Governance is a system for defining, within an organization, who has authority and control over data assets and how they can be used. It encompasses the people, processes, and technologies needed to manage and protect data assets.
As companies adapt to new data privacy regulations and rely more on data analytics to optimize processes and operations to improve business decisions, the process becomes more complicated. Data Governance ensures that data is consistent, reliable, and not misused.
Without good data governance, customer data may appear differently in sales, logistics, and customer service systems, complicating data integration efforts.
Its principles are as follows:
- Transparency: members of an organization must be clear about the data assets they have to share this knowledge with staff and customers, noting what data is collected, its purpose, where it is stored, and with whom it interacts.
- Accountability: Everyone in an organization should be aware that they are responsible for the data they deal with, not just the IT department. Although the responsibility may lie with one or the other, a common and responsible mindset must be implemented.
- Integrity: We must ensure that the data we store is relevant, accurate, and compliant with security policies and regulations. Therefore, we must establish a culture of honesty around data policies and consolidate processes to ensure data quality.
- Collaboration: It is essential that departments can talk and discuss best practices for processing data in different business areas. By collaborating across teams, practical data standards can be established to improve data governance.
Data Governance Best Practices
Good data governance is not a simple task, as it requires teamwork, investment, resources, planning, and monitoring.
Data Governance strategies must be tailored to a company’s processes, needs, and objectives. For this reason, there are five fundamental best practices to follow:
- Identify critical data elements and treat them as a strategic resource.
- Establish policies and procedures for the entire data lifecycle.
- Involve business users in the governance process.
- Do not neglect the management of data masters.
- Do not overly restrict the use of data.
Data Governance vs. Data Management
Data governance is only one part of the overall data management discipline, albeit very important.
While data governance refers to the roles, responsibilities, and processes for ensuring accountability and ownership of data assets, data management is defined as an umbrella term that describes the processes used to plan, specify, enable, create, archive, control, and purge data.
In other words, you can manage data without data governance policies, but not vice versa.
Governance addresses who owns what data and who can access it, as well as whether X data is subject to privacy laws or other regulatory requirements and what its security requirements should be.
Good data governance ensures the effective and efficient use of data assets, which translates into achieving a company’s KPIs by defining the data workflows or people processes that provide the necessary data quality and security.
Data Governance Implementation
We have already looked at data governance best practices, but implementing them is a much harder task. Every organization is different, so you must apply an agile development mindset, starting with an MVP and then iterating to grow. This will help generate greater long-term benefits and make it easier for the organization to align with the established guidelines.
Think about the big picture, but start small
Since data governance is a combination of people, processes, and technology, to start building the big picture, it is best to start with people. Then, we can move on to developing the processes and finish by incorporating technology.
We must be clear that, without the right people, it will be very difficult to build successful processes for the technical implementation of data governance.
Start with a business case
You will need to create a solid business case that shows the benefits and opportunities that good data quality will bring to the organization, as well as the improvements that can be made (increased revenue, better customer experience, efficiency, etc.).
This will help all parties involved to see and better understand the efforts they must invest to achieve the benefits that will lead to success.
Measure everything
Like any other objective, if it can’t be measured, it can’t be achieved and doesn’t exist.
When making any change, you should collect metrics in advance and then constantly track each step throughout the implementation of this new strategy. Metrics will be your best ally to show changes and improvements over time and serve as checkpoints to ensure practical and effective processes.
Think of this process as a marathon, not a sprint
Athletics metaphor aside, good data governance has no finish line because it is a long-term investment. It is not a project with a start and end date.
Data governance is an ongoing, iterative process with many sub-projects and milestones. You may start with small pilot tests and then take the learnings to the rest of the enterprise to launch more significant, more comprehensive initiatives.
Data Governance requires teamwork, the results of which will be seen in all departments. Clearly defining the roles involved and assigning ownership levels in the organization is essential.
Determining who has authority and responsibility will help put your data governance program in place and establish a smart structure to address data programs with strength.
These may include a governance council (strategic level), data governance board (tactical level), stewards, owners, users, etc. Each organization is unique, and these levels may vary to include more or fewer roles.
Why does a company need Data Governance?
Many companies already have some form of governance in place for applications, business units, or individual functions. However, formal and systematic control over these processes and responsibilities must be established.
Opting for this strategy can help companies remain competitive, especially as they grow to a size where it is no longer efficient for people to perform cross-functional tasks.
In fact, according to Gartner’s D&A Governance survey, 61% of companies said their governance goals included optimizing data for business processes and productivity. Still, only 42% of that group believed they were on track to achieve it. The survey also predicts that, by 2025, 80% of organizations looking to scale digital business will fail because they do not adopt a modern approach to data governance.
At Plain Concepts, we help you adopt a “data product” mindset so you can incorporate collaboration into workflows, as well as adopt DataOps to leverage customizable and programmable tools.
We’ll start by identifying the use case that best fits you to achieve maximum ROI and get you to scale data governance for the remaining data use cases.
We help you discover how to get value from your data, monitor and analyze all your data sources, and use data to make smart decisions and accelerate your business:
- Data analytics and strategy assessment: we evaluate data technology for architecture synthesis and implementation planning.
- Modern analytics and data warehouse assessment: we provide you with a clear view of the modern data warehousing model through understanding best practices on how to prepare data for analysis.
- Exploratory data analysis assessment: we look at the data before making assumptions so you get a better understanding of the available data sets.
- Digital Twin and Smart Factory Accelerator: we create a framework to deliver integrated digital twin manufacturing and supply chain solutions in the cloud.
We will be your best ally in your journey to become a data-driven company. Start getting value and performance from your data today!