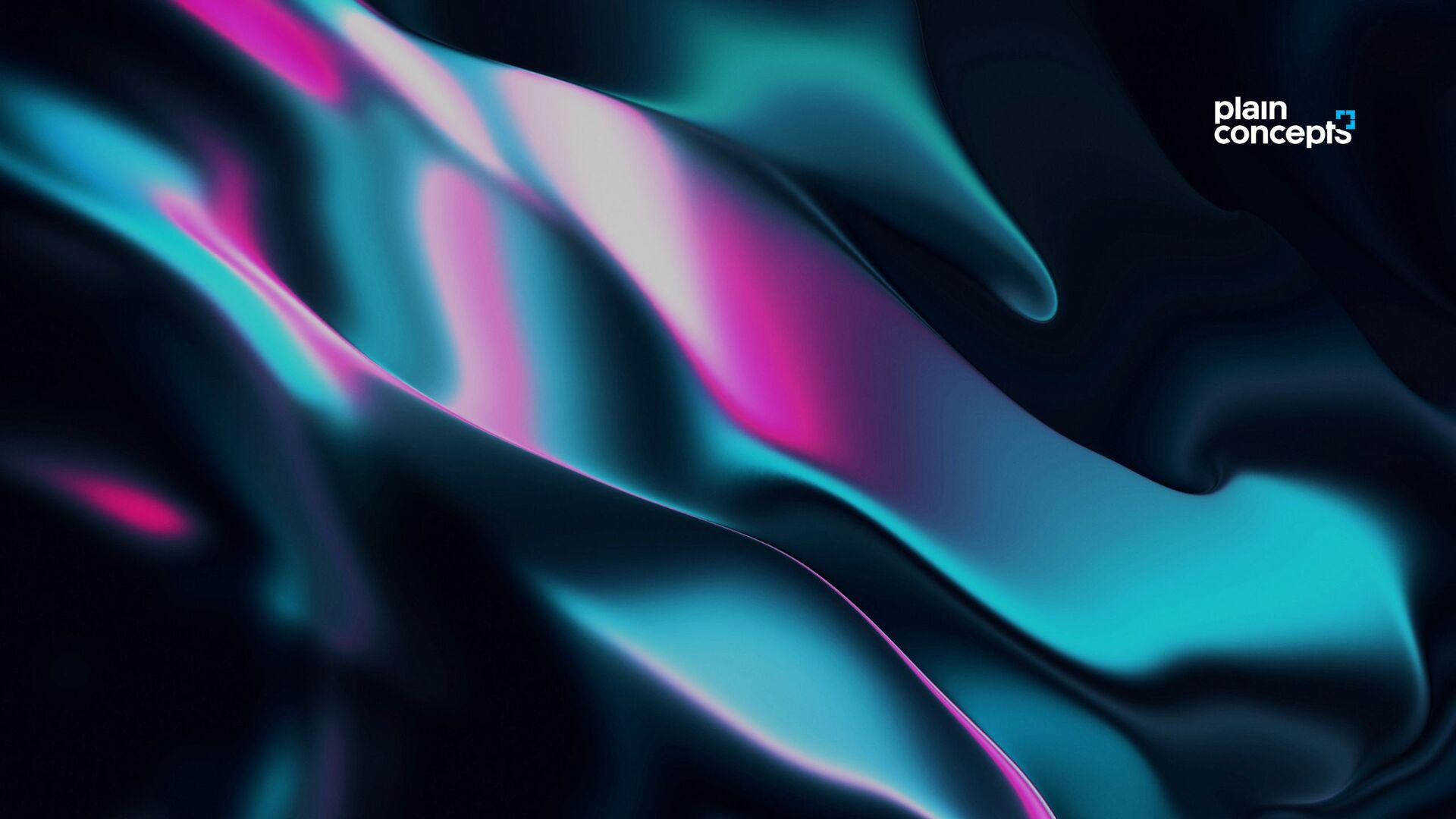
Discriminative AI vs Generative AI: Keys to understanding them
Deep Learning has become one of the key technologies for unlocking the full potential of AI in various domains. Its two main approaches encompass generative and discriminative models.
Distinguishing one from the other can be misleading, so we break down the differences by explaining their capabilities, characteristics, and approaches.
General Approach
Traditional or discriminative AI is based on three pillars: supervised, unsupervised, and reinforcement learning, which provides information based on the outcome of clustering.
Generative AI refers to systems that can generate new content (text, images, music, videos).
Approach
Non-generative AI models perform computations based on input data (such as classifying an image or translating a text into another language).
In contrast, generative AI models produce “new” results, such as writing texts, composing music, designing images, etc.
Goal
Generative models delve into the underlying distribution of the input data. By understanding the joint probability distribution of this data and labels, they can generate content that resembles the training data, allowing for creative data synthesis and augmentation.
Discriminant AI, on the other hand, instead of modeling the entire data distribution, focuses on capturing the conditional probability distribution of the labels based on the input data.
Training Focus
Generative models take advantage of unsupervised learning techniques to unravel the underlying distribution of data. They take advantage of unlabelled data and try to capture the complex patterns and structures present in it. Therefore, the training techniques for these models include maximum likelihood estimation, variational inference, and adversarial training.
Discriminative models are based on supervised learning, using labeled data where both input data and labels are available during training. They focus on minimizing classification errors or maximizing the probability of accurate class predictions. They employ techniques such as backpropagation, gradient descent optimization, and regularisation to adjust the decision boundary and obtain optimal classification accuracy.
Data Generation
By capturing the underlying distribution, generative models can generate new samples as training data. They can create new instances with similar characteristics to the original dataset, opening up opportunities for data augmentation or image synthesis.
Discriminative models do not have inherent data generation capabilities, but they can indirectly generate new samples using data augmentation techniques. This involves applying transformations to existing data, such as rotations, translations, or rotations, to extend the diversity of the training set.
Decision Limit
Generative models indirectly capture the decision boundary by modeling the underlying data distribution. By understanding data patterns and relationships, they can generate samples from various regions of the feature space, even in regions where training data may be sparse.
Discriminative models excel at explicitly learning the decision boundary between classes. Instead of modeling the entire data distribution, they optimize the decision boundary to achieve accurate classification. As such, they work very well when the boundary is relatively simple or when classes are well separated.
Generative and discriminative models offer unique and distinct advantages and applications. Understanding their differences is crucial to be able to take advantage of their potential, therefore, having an expert team in the field will be the differentiating factor to ensure success.
At Plain Concepts, we create 360º AI solutions, accompanying you in defining and implementing the AI adoption strategy in your company. We have our own AI R&D team to find innovative ways to apply artificial intelligence in business, devising new disruptive uses for more than ten years.
To tackle an AI-assisted strategy, you need to solve technological, informational, cultural, and organizational challenges. That’s why we define the strategy that best suits you gradually and with tangible benefits.
If you want to implement this technology in your business, don’t wait any longer and contact us!