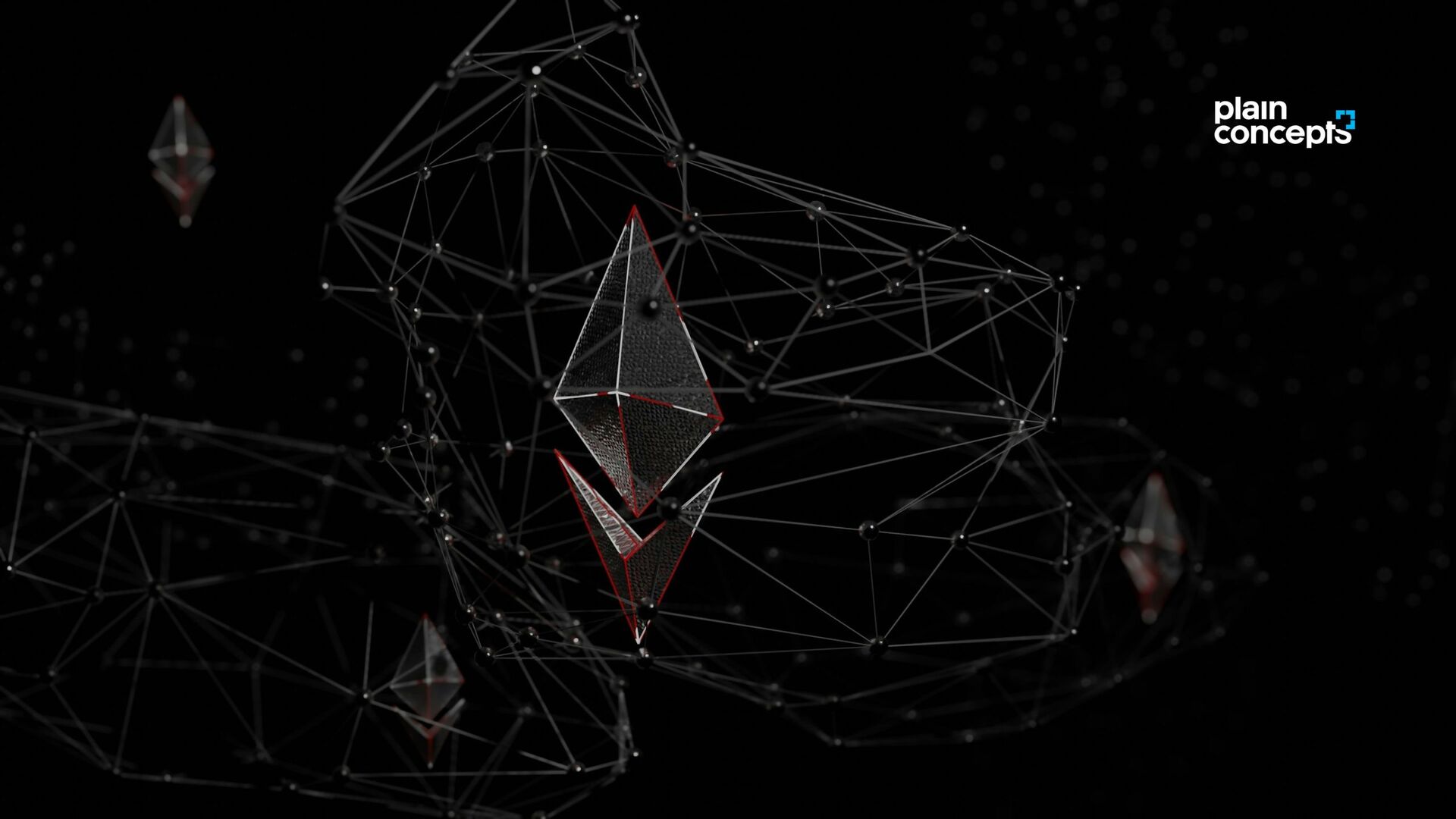
The present and future of Data Architecture: The evolution of the data-driven enterprise
Although overlooked by many companies, data architecture is one of the most important facets of the enterprise technology landscape. Over the past few years, it has been gaining importance and transforming itself, driven by AI, Big Data, and an increased demand for real-time data processing.
Data trends and forecasts for the future of data architecture are showing momentum, promising to redefine the way we manage data and the way business decisions are made.
With new trends such as real-time data processing, the data grid, and advanced analytics, data architecture is set to become a key transformative and disruptive factor. We’ll tell you all about it!
Evolution of Data Architecture
Data architecture is evolving to deliver metadata-enabled data self-service.
Best practices for efficient data analytics architecture have evolved over the past decades, driven by digital transformation and the need to modernize strategies and leverage data for greater business benefit.
According to Gartner, the chronological distribution would look like this:
- Before 2000 and the Enterprise Data Warehouse (EDW) era: architecture focused on the success of these data warehouses.
- 2000 to 2010 and post-EDW: In this period, there was a strong focus on the analysis of fragmented data, where there was a reliance on data warehouses. Each data mart consolidation led to another data silo that generated fragmented and inconsistent analysis.
- 2010 to 2020 and the era of the logical data warehouse (LDW): the move to more unified data analysis through a common semantic layer, enabling access to data warehouses, data marts, and data lakes. This is still the most common practice.
- 2020 onwards: over the next few years, we will see augmented data analytics using all relevant data sources, accessed and enabled by advanced analytics, recommendation engines, data orchestration and artificial intelligence, adaptive practices, and metadata analysis.
The democratization of data access and self-service analytics is driving the evolution to an era of active metadata. These will extend the use cases beyond those facilitated by LDW, from master data management, business-to-business data exchange, B2B data integration, partner data exchange, application data integration, etc.
This metadata describes different facets of the data, as if it were a by-product of the movement of data through business systems. There are four types: technical, operational, business, and social. Each type can be ‘passive’ metadata that organisations collect, but do not actively analyse, or ‘active’ metadata that identifies actions in two or more systems that use the same data.
Data Fabric
Data Fabric is an emerging concept of data integration and data management design, which aims to achieve flexible, reusable, and augmented data integration to support data access across the enterprise.
Data architecture has been a natural evolution for enterprises, from their logical storage models to modernizing the architecture by leveraging technology and metadata.
This approach offers numerous benefits from different perspectives:
- Enterprise: Enables less technical users to find, integrate, analyze, and share data quickly.
- Data management team: gain productivity benefits from automated data access and integration, leading to greater agility and better results.
- Organization: businesses gain faster insights from investments in data and analytics, improve organizational data utilization, reduce costs, and gain more insight into the design, delivery, and effective use of data.
The factors that determine whether the design of the data structure is appropriate for each case are the integrity of the metadata and the expertise in a data structure within the organization. Those with little or no metadata will find it very difficult to find benefits and will see an increased reliance on experts to help discover, infer, or create metadata.
Data Mesh
The data grid adopts an architectural approach that enables decentralized data management. It aims to define, deliver, maintain and govern data products in a way that makes them easy to find and use by data consumers.
Thus, the Data Mesh architecture is based on decentralizing and distributing responsibility for data to those closest to the data and sharing it as a service.
It offers numerous benefits, such as greater autonomy for lines of business, less dependency on central IT, and breaking down silos by decentralizing data.
The success of this architecture will depend on the organizational model and data skills in the business lines. How does this work? If data literacy, autonomy, and data skills are not consistent and vary greatly by department or organization, strategies can be put in place; central IT will be more supportive.
Properly implemented, lines of business can evolve towards greater autonomy within a data grid environment by creating new roles to manage the definition, creation, and governance of data products. Provided they are committed to developing distributed data skills.
The data mesh shifts responsibility for data governance to domain application designers and users, so for a line of business to autonomously create and expose data products, it must define local data governance and management that complies with central guidance from the CISO and CDO.
This is a good option for organizations with incomplete metadata. Provided they have experienced data architects who can start with this architecture and build active metadata stores in parallel.
Flexible Architecture
Flexibility is one of the most sought-after characteristics in any organization that is in the midst of digitization and wants to overcome the challenges of the complexity of modern environments.
At a time when we are working with on-premises, cloud, multi-cloud, hybrid, etc., companies need to review their strategy for a robust and planned data architecture. This is the only way to ensure that the new technologies implemented will be consistent with the existing infrastructure and will be able to support future demands.
The key to achieving this lies in 4 pillars:
- Design a strategy that encompasses the entire data ecosystem: It is crucial to establish an overall cloud strategy that prioritises providers’ ability to make additional deployments. This mitigates the risks that unauthorized clouds may pose to the architecture.
- Align data requirements with use cases: Distributed and complex use cases drive new innovations that deliver business value and self-service data access. Success will depend on the ability to meet the use cases of business consumers.
- Evaluate integration patterns: The challenge of moving data across different on-premises and cloud systems, as well as the rapid volume growth, makes it critical to evaluate patterns to identify a reliable and efficient architecture that serves evolving business use cases and addresses sovereignty and compliance needs.
- Adoption of open source and standards for future investments: Using open or neutral standards, as well as understanding storage options and open source standards make metadata shareable across platforms in an enterprise environment.
Enterprise Data Architecture
According to McKinsey, there are three key factors that are driving economic growth in reference to data: competitive advantages for technology and data-driven companies, higher returns for companies that continuously invest in innovation, and the economic potential of generative AI for the creation of new use cases.
All of this demonstrates the importance of data as a driver of this knowledge, without which all other technologies cannot function properly. This is why it is so important for decision leaders to move towards a data-driven model. To do this, some changes can be implemented in the organization, such as:
- Prioritise quality data: It is important to move from a sporadic and inefficient data-driven approach to a model where data is harnessed, and innovative techniques are used to solve challenges.
- Leverage data and analytics technology: Typically, only a small fraction of the data available in the enterprise is ingested, processed, and analyzed in real time due to the limits of legacy systems. Moving to sophisticated advanced analytics models (generative AI, self-service data, and low-code platforms) will be key to generating faster and more powerful insights.
- Create dynamic and reusable data products: Data engineers often spend a lot of time repeatedly selecting and debating data sets. However, selecting rich, multi-purpose, dynamic data products minimizes data engineering and facilitates high-impact use cases, maximizing time to market and ROI.
- Treat data as a product: Data sets are often stored in isolated, expensive, and unowned environments. However, treating it as a product with teams aligned with it to build in security, develop engineering, and implement self-service access and analytics tools will help meet the needs of all users.
- Expand the role of the chief data officer to generate value: Rather than giving them the role of developing and tracking compliance with policies and standards, CDOs and their teams should devise ways to use data, develop a business strategy and incubate new revenue streams to monetise services.
- Ecosystem integration as the norm: Instead of having data in isolation and having difficulty sharing, there should be a shift to data-sharing platforms to facilitate project collaboration. This will actively participate in a data economy to create more valuable user insights.
- Prioritise and automate data management: Many companies view data management, privacy and security as compliance issues that are governed by manual processes that make it difficult to retrieve and protect data. AI tools and innovative techniques can now be used to enable self-describing data, improve quality and generate scripts that provide secure access to data in near real-time.
Changes such as those mentioned above will have very positive results in the organisation. However, many companies fail to translate these best practices into concrete and tangible actions. Change must be broad and deep, starting with the development of a clear roadmap that prioritises the value domains and identifies the data sources that can drive the necessary solutions.
Modern Data Architecture
The design of the data architecture is fundamental to creating a vision of the interactions that occur between data systems. It also describes the type of structure to manage data in a simple way and facilitate data pre-processing.
At Plain Concepts we help you define your business requirements and policies, technologies in use, economics and data processing needs to manage them efficiently through an architecture that will help you achieve your goals.
Our aim is to approach the challenge of digital and data strategy from a business perspective that will deliver benefits using a structured framework that meets your needs.
With this approach, we define the necessary digital and data strategy through immersion, maturity, and consolidation, working on generating short-term benefits that give credibility to this strategy.
- We assess the company’s data maturity level.
- We identify the critical data to manage, control, and exploit.
- We establish objective use cases, focus on generating medium-term benefits, and design the initiatives to implement them.
- We generate interest and commitment in your team through training on the importance and potential of data-driven management.
If you want to start turning your data into actionable information with the latest data architecture, storage, and processing technologies, contact our experts and start your transformation now!