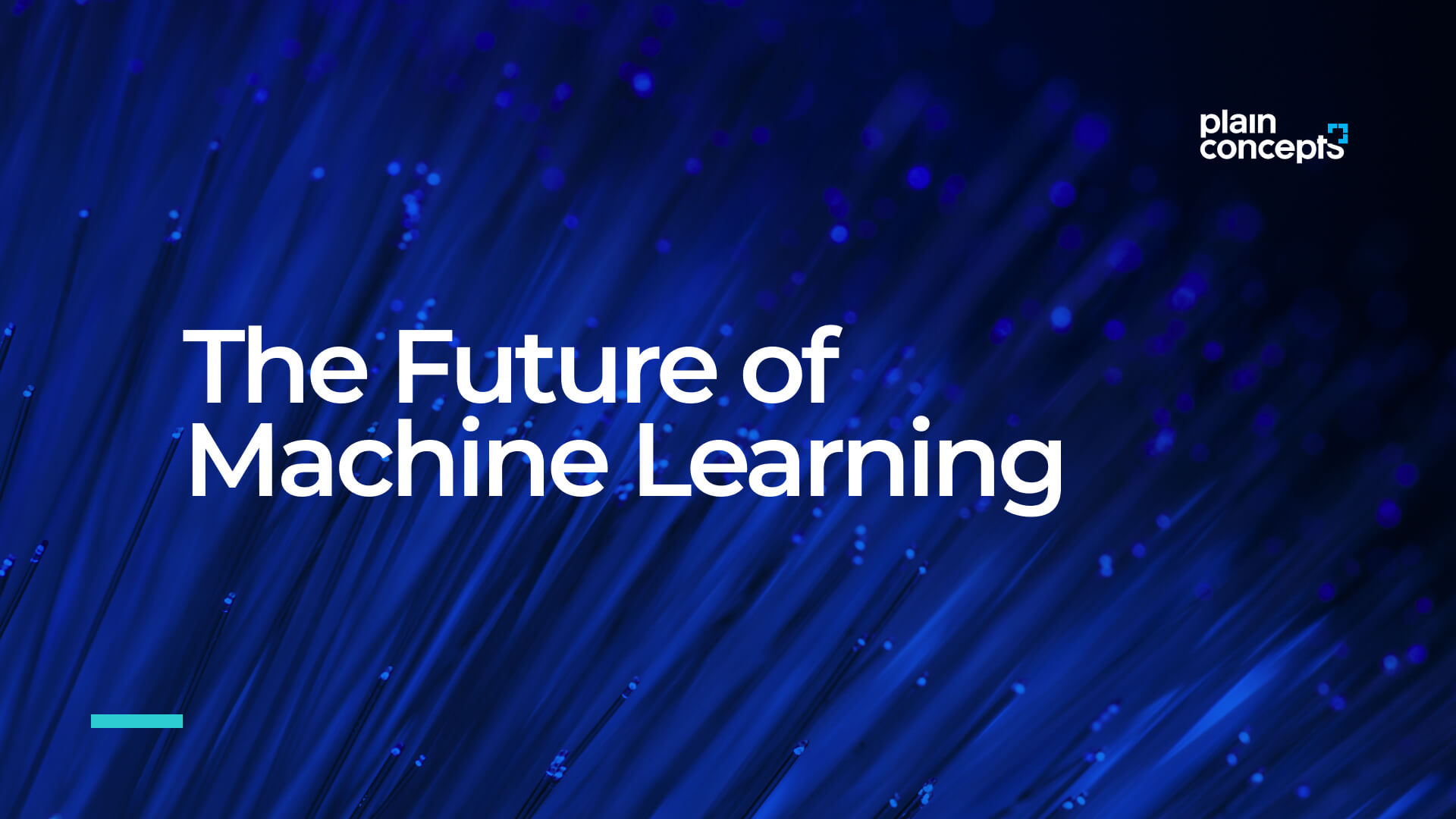
Machine Learning for the business of the future (and the present)
Machine Learning is so versatile and offers so many applications that it has become one of the most critical technologies. It has become crucial in companies, enabling entrepreneurs to understand customer behavior and business performance better.
Leading companies in various industries are already using this technology effectively and have made it a critical operational part of their processes. We analyze the future of Machine Learning (ML) and its value in different sectors.
What is Machine Learning?
Machine Learning is a branch of artificial intelligence that relies on data and algorithms to replicate how humans learn, gradually improving its accuracy as it is trained.
The term was first used in 1959, but its relevance has been growing thanks to advances in computing capabilities and big data over the past few years.
It is an essential component in data science, a growing, and increasingly important area. Using statistical methods, algorithms are trained to make classifications or predictions and thus extract decisive information. It is this information that subsequently drives decision-making within businesses.
ML endows computers with the ability to learn independently and perform tasks autonomously without needing to be constantly supervised by a human. The result is autonomous cars, assistants translating in real-time from one language to another, or personalized shopping and content suggestions on streaming platforms.
Current Machine Learning landscape
Machine intelligence and machine learning make up a $14.1 billion industry transforming how we understand and use data.
According to a study by Helomics, the global AI market is expected to grow to $20 billion by 2025. This represents a huge growth opportunity for businesses and disruption for industries.
It enables business leaders to make decisions based on more helpful information and analyze problems in new ways, making it the best ally for humans now and in the future.
Joining this revolution is, therefore, a vast market opportunity. Artificial intelligence is becoming a significant part of many businesses, and ML adoption plans are accelerating faster and faster, especially after the impact of COVID-19 on companies and workplaces worldwide.
Machine Learning vs. Deep Learning
Doubts about the differences between Machine Learning and Deep Learning may arise, so let’s analyze them.
We must start from the fact that both are types of artificial intelligence, and ML can adapt automatically with minimal human intervention. DL is a subset of machine learning that uses artificial neural networks to mimic the human brain’s learning process.
But these are not the only differences:
Machine Learning Applications
ML is one of the pillars underpinning digital transformation, so it is being used to find new solutions in different areas, such as:
- Intelligent vehicles: thanks to ML, cars can adjust internal settings according to the driver’s preferences or move the steering wheel to react to the environment.
- Personalized recommendations: this allows you to make customized suggestions on online platforms. It analyzes the user’s history and visits or compares them with others to identify trends or similar habits.
- NPL: by understanding human language, assistants can translate from other languages, recognize the user’s voice or analyze their feelings. Natural language processing can also be used for more complex tasks, such as classifying large volumes of information or helping to translate legal jargon into plain language.
- Cybersecurity: today’s antivirus and malware detection engines use ML to boost scanning, accelerate anomaly detection and improve responsiveness.
- Medicine: many research centers are already using Machine Learning to detect diseases such as breast cancer early, which is crucial to increase the chances of curing it. It is also beneficial for detecting pneumonia or eye diseases.
- Social networks: machine learning is very effective in reducing spam or detecting fake news and unauthorized content, even in live broadcasts.
- Computer Vision: allows meaningful information to be obtained from digital images, videos, and other visual inputs to make the right decision. It can be applied for photo tagging in social networks, medical X-rays, or autonomous cars.
- Fraud detection: detecting suspicious transactions is very useful in the banking and financial sector. A model can be trained using information about known fraudulent transactions to identify anomalies that merit further investigation.
- Predictive equipment maintenance: uses data-driven techniques to monitor equipment condition and predict potential failures before they occur.
Future of Machine Learning
ML offers growth opportunities and revolutionizes numerous industries by enabling more informed decisions, helping developers analyze problems with an innovative approach, and delivering information faster and with greater accuracy.
As a result, the future of machine learning is full of possibilities. Today, machine learning applications can nourish almost every common domain, so it is easier to imagine a lot with it.
Advances in NPL and Computer Vision are accelerating its applications in facial recognition, language translation, autonomous cars, etc. What seemed like science fiction is now a reality.
Now, the next step will go hand in hand with quantum computing, as ML has the potential to transform and innovate thanks to quantum algorithms. It will be possible to improve data analysis and gain deeper insights. The result of better performance is going to help companies achieve better results than with more traditional machine learning methods.
Currently, there is no commercially ready quantum Machine Learning model, but large companies are already investing in this technology so the wait will be short.
It is also expected that a multi-use model will be created over the next few years to perform several tasks simultaneously. No longer will there be a need to worry about understanding the relevant applications of a framework, but rather a model will be trained on multiple domains as needed.
In addition, with the proliferation of databases and cloud storage, data teams are asking for more flexibility when using data sets across multiple systems. As a result, there will be significant advances in distributed machine learning, where data scientists will no longer reinvent algorithms from scratch for each platform. They will be able to immediately integrate their work into the new systems and experience model portability.
Finally, another forecast is reinforcement learning (RF), which allows companies to make intelligent business decisions in a dynamic environment without being specifically taught to do so.
Machine Learning Solutions
The automation of decisions assisted by ML models is covering more and more applications and business levels. As such, the importance of MLOps models lies in the fact that they are vital to streamlining the maturation process of AI and ML projects within an organization.
At Plain Concepts, we help companies manage their ML projects by providing expert guidance on MLOps, including assessing current capabilities and applying industry standard practices to maintain a production-ready ML environment.
If you are ready to start or advance your project, we can assess your current level of maturity through a preliminary assessment report – get your MLOps report!