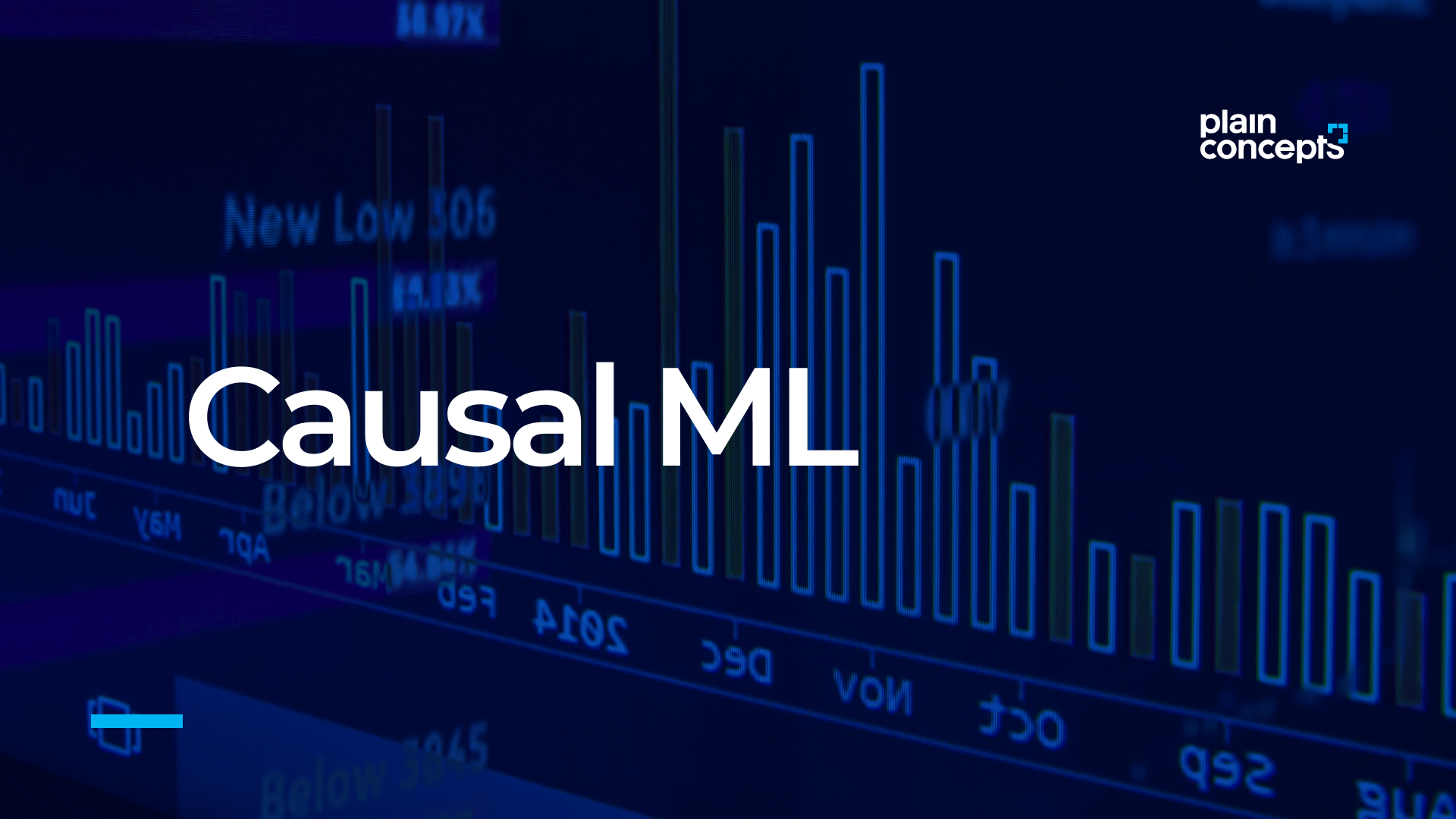
Causal ML: What is it and what is its importance?
Artificial Intelligence is currently on everyone’s lips. There are more and more processes that somehow include some kind of Machine Learning or Deep Learning model to achieve a positive impact on their business processes. All of them are trained on data to obtain the relationships and give a prediction on these. However, this highlights the problem that correlation does not imply causation. For example, we can try to compare the number of PhD graduates per year in Computer Science with the total profit generated by arcade machines.
Figure 1: Spurious correlations
As we see in the image, both show a high correlation. A model in this case could extract the correlation of both variables, but we have a spurious correlation. Since the model does not understand the causes behind the relationships between the data, it makes it necessary for us to understand the question of why these relationships exist. And it is here that a little-known science is born, although it is also increasingly on the rise, such as causal inference.
Causal inference
Causal inference is a fascinating and useful topic in many fields. It is the process of identifying and understanding cause-effect relationships between variables. In many areas of research, including epidemiology, economics, political science, and psychology, causal inference is crucial to understanding the effects of interventions, policies, or programs. Causal inference also helps predict the outcome of changes in variables, which can be especially useful in the design of experiments and in decision-making.
Causal inference is the process where causes are inferred from data and on any type of data as long as there is enough of it, so causality is about interventions, about doing. Whereas standard statistics is about correlations, but they can lead to erroneous assumptions that lead to much worse things. If we start to formalize the use of causality, it is about inferring a treatment or a policy T from an outcome Y.
But this is best seen with an example.
Sleeping in shoes causes headaches.
If we look for a reason or cause for this behavior, it could be that we had been drinking the night before.
This reason is called confounding variable. This variable is related to both the input variable and the output variable in a model and can distort the causal relationship between them. In other words, confounding is a variable that affects both the input variable and the output variable and can give rise to an apparent causal relationship that is actually spurious. Finally, the total association would be:
Formally, T causes Y if a change in T causes a change in Y, and holds all else constant. Then, the causal effect is the magnitude by which Y changes by changing one unit in T:
Causal ML
So far we have only explained what causality is all about, but you may be wondering, “How the heck can I apply causation on my ML model?”. For this comes Causal ML, an emerging area of research that seeks to improve the ability of machine learning models to capture causal relationships in data. Causal inference in machine learning is based on the idea that correlations between variables are often not sufficient to establish causal relationships, as there may be other variables that influence both.
Machine learning models often rely on correlation learning, i.e., the ability to find patterns in data to make predictions. However, this capability can be limited in situations where a deeper understanding of the underlying causal relationships is required. Causal inference in machine learning seeks to address this limitation by using techniques and algorithms that take into account the causal relationships between variables.
The goal of causal inference in machine learning is to improve the accuracy and interpretability of models, which can have important implications in areas such as health, economics, policy, and justice. For example, causal inference models can be used to understand the effects of interventions and policies, to control for biases in data, and to provide greater transparency and explainability in automated decisions.
In summary, causal inference in machine learning is an important area of research that seeks to improve the ability of machine learning models to capture causal relationships in data. This capability may have important implications in a wide variety of fields, and causal inference in machine learning is expected to continue to be an active area of research in the future.
What Causal ML can help you with
Now that we have introduced Causal ML, it is important to know what we can use this technology for.
Causal ML tries to identify the causes underlying the data, so it can be a technique to increase the personalization of our users. On the other hand, by understanding why a product is used, we can better target it to different individuals.
Thus, some interesting use cases can be:
- Improving marketing decision-making: causal inference can be used to assess the impact of marketing campaigns on customer acquisition or on the loyalty of existing customers. Thus, by understanding the causal relationship between marketing actions and business results, strategies are optimized and ROI is improved.
- Operational process optimization: can be applied to operational processes such as manufacturing or logistics. By understanding the causal relationships between key variables, bottlenecks and areas for improvement in processes can be identified, leading to significant improvements in efficiency and quality.
- Fraud prevention: by analyzing the causal relationships between key variables, suspicious patterns indicating fraud or illegal activities can be identified.
If you have been attracted by the advantages of Causal ML, we work with you to bring out its full potential.